The Future of AI Development: Trends, Challenges, and Opportunities
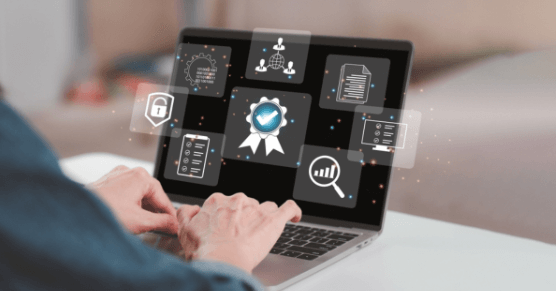
Artificial Intelligence (AI) is transforming various sectors, revolutionizing how businesses operate and innovate. From healthcare and finance to autonomous vehicles and retail, AI is powerful efficiencies, enhancing decision-making, and creating new opportunities for growth. Its ability to process vast expanses of data, recognize patterns, and make likelihoods is leading to smarter, more responsive systems across industries.
The growing interest in AI research, development, and deployment is operated by its potential to solve complex problems and optimize processes. Governments, academia, and set apart enterprises are heavily investing in AI development services to stay competitive and come across the evolving demands of the digital age. This surge in interest is paving the way for groundbreaking advancements and practical applications that are reshaping our world.
Trends Shaping AI Development
Ethical AI
Ethical considerations are crucial in AI development to ensure that technology benefits all of society fairly. This includes mitigating partialities that can lead to unfair treatment or discrimination, ensuring transparency in AI decision-making processes, and stimulating fairness. Developers and scientists are increasingly focusing on creating ethical AI frameworks, such as those discussed onĀ www.talkie-ai.com, that prioritize these aspects, ensuring that AI systems are trustworthy and equitable.
Explainable AI (XAI)
As AI models become more complex, the need for interpretability and clearness has grown. Explainable AI (XAI) aims to make AI models understandable to humans, allowing stakeholders to see how pronouncements are made. This is particularly important in sectors like healthcare and finance, where understanding the rationale behind an AI’s decision is life-threatening. Techniques such as visualizations, simplified models, and rule-based systems are being developed to make AI more interpretable.
Edge AI
Edge AI involves processing data closer to its source, such as on local devices rather than centralized data centers. This trend is driven by the need for real-time decision-making and reduced latency, above all in applications like autonomous vehicles, IoT devices, and smart cities. By moving AI processing to the edge, organizations can achieve faster responses and improve the efficiency of their systems.
Federated Learning
Federated learning is revolutionizing AI by enabling collaborative model training across distributed devices while preserving data privacy. Instead of aggregating data into a central server, federated learning allows models to be trained locally on individual devices. The locally accomplished models are then aggregated to form a global model. This approach enhances privacy and security, as raw data never leaves the local device, making it ideal for applications in healthcare, investment, and other sensitive fields.
Quantum AI
Quantum AI explores the potential of quantum computing to solve complex AI problems that are currently beyond the reach of classical computers. Quantum computing leverages the principles of quantum workings to process information in fundamentally different ways, offering exponential speed-ups for certain types of calculations.
Challenges Ahead
Data Quality and Bias
One of the significant challenges in AI development is ensuring the quality and fairness of training data. Biased data can lead to prejudiced AI models, which can perpetuate and even enlarge prevailing inequalities. Addressing these issues requires diligent data collection, preprocessing, and continuous monitoring to ensure that datasets are representative and free from bias. High-quality, diverse datasets are crucial for training reliable AI systems.
Scalability
Deploying AI models at scale presents numerous challenges, particularly in terms of computational possessions and infrastructure. AI models every so often require significant processing power and storage, which can be difficult to manage as the volume of data and complexity of models grow. Scalability issues also include maintaining performance and accuracy as models are applied to larger datasets and broader applications. Solutions involve heightening algorithms, leveraging cloud computing, and employing distributed systems.
Interoperability
As AI technologies proliferate, the need for standardized interfaces and interoperable systems becomes critical. Different AI tools and platforms often have unique protocols and formats, creating silos and hindering integration. Establishing industry standards and promoting interoperability make sure that AI systems can seamlessly communicate and work together, enhancing their utility and efficiency. This is especially important in multi-system environments like smart cities and integrated healthcare networks.
Regulatory Landscape
The regulatory environment surrounding AI is rapidly evolving, with governments and organizations worldwide developing frameworks to govern AI development and deployment. These regulations purpose to address ethical concerns, data privacy, and security issues. Staying abreast of these changes is essential for compliance and to avoid potential legal pitfalls. Navigating this landscape requires a proactive approach to understanding and implementing regulatory requirements in AI projects.
Opportunities for Innovation
Healthcare
AI is revolutionizing healthcare with applications in disease diagnosis, drug discovery, and personalized medicine. AI algorithms can analyze medical images, predict disease outbreaks, and assist in developing new treatments. Made to order medicine, which tailors healthcare to individual patients based on their genetic makeup and lifestyle, is another promising area where AI is making significant strides.
Autonomous Systems
Advancements in AI are driving the development of autonomous systems, including self-driving cars, drones, and robotics. These technologies promise to transform industries by enlightening safety, efficiency, and convenience. For example, self-driving cars can reduce traffic accidents, drones can heighten delivery services, and robotics can automate complex manufacturing processes.
Natural Language Processing (NLP)
Natural Language Processing (NLP) is enhancing human-computer interactions through chatbots, virtual assistants, and language translation services. NLP technologies enable machines to understand and respond to human language, making them invaluable for customer service, content creation, and real-time translation. These applications improve user experience and operational efficiency across various sectors.
Climate Change Solutions
AI is playing a crucial role in addressing climate change by enabling better environmental monitoring, climate modeling, and sustainability efforts. AI can analyze vast amounts of environmental data to predict climate trends, optimize energy consumption, and manage natural resources more effectively. These innovations support efforts to mitigate the impacts of climate change and promote sustainable practices.
Conclusion
Staying informed about AI trends and addressing associated challenges is essential for leveraging the full potential of AI. The sprouting landscape of AI presents both challenges and opportunities that require continuous research, collaboration, and responsible development practices. At Hashlogics, we are committed to navigating these complexities and inventive innovative AI solutions that drive progress and sustainability. By embracing AI solutions by Hashlogics thoughtfully and ethically, we can unlock new possibilities and contribute to a better future.